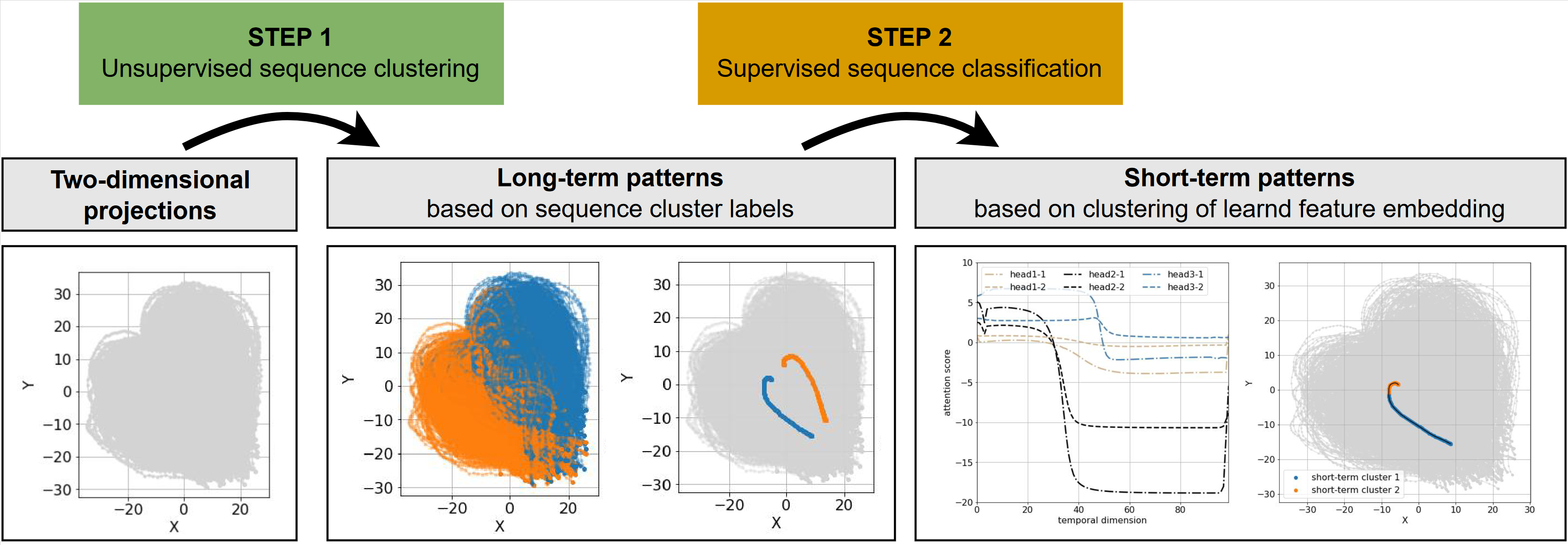
Abstract
Combining explainable artificial intelligence and information visualization holds great potential for users to understand and reason about complex multidimensional sequential data. This work proposes a semi-supervised two-step approach for extracting long- and short-term patterns in low-dimensional representations of sequential data. First, unsupervised sequence clustering is used to identify long-term patterns. Second, these long-term patterns serve as supervisory information for training a self-attention-based sequence classification model. The resulting feature embedding is used to identify short-term patterns. The approach is validated on a self-generated dataset consisting of heart-shaped paths with different sampling rates, rotations, scales, and translations. The results demonstrate the approach's effectiveness for clustering semantically similar paths and/or path sequences. This detection of both global long-term patterns and local short-term patterns facilitates the understanding and reasoning about complex multidimensional sequential data.
Citation
Matthias Bittner,
Andreas
Hinterreiter,
Klaus
Eckelt,
Marc
Streit
Explainable Long- and Short-term Pattern Detection in Projected Sequential Data
ECML PKDD Workshop on Explainable AI for Time Series: Advances and Applications (XAI-TS '23),
2023.
BibTeX
@inproceedings{2023_pattern_detection_xai, title = {Explainable Long- and Short-term Pattern Detection in Projected Sequential Data}, author = {Matthias Bittner and Andreas Hinterreiter and Klaus Eckelt and Marc Streit}, journal = {ECML PKDD Workshop on Explainable AI for Time Series: Advances and Applications (XAI-TS '23)}, year = {2023} }