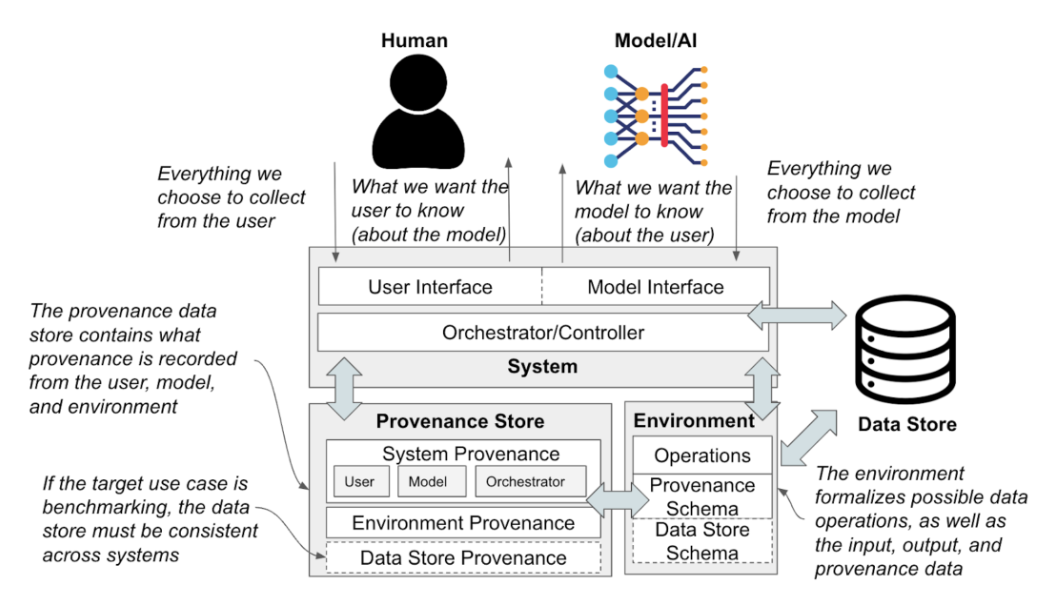
Abstract
The scope of automated machine learning (AutoML) technology has extended beyond its initial boundaries of model selection and hyperparameter tuning and towards end-to-end development and refinement of data science pipelines. These advances, both theoretical and realized, make the tools of data science more readily available to domain experts that rely on low- or no-code tooling options to analyze and make sense of their data. To ensure that automated data science technologies are applied both effectively and responsibly, it becomes increasingly urgent to carefully audit the decisions made both automatically and with guidance from humans. This Dagstuhl Seminar examines human-centered approaches for provenance in automated data science. While prior research concerning provenance and machine learning exists, it does not address the expanded scope of automated approaches and the consequences of applying such techniques at scale to the population of domain experts. In addition, most of the previous works focus on the automated part of this process, leaving a gap on the support for the sensemaking tasks users need to perform, such as selecting the datasets and candidate models and identifying potential causes for poor performance. The seminar brought together experts from across provenance, information visualization, visual analytics, machine learning, and human-computer interaction to articulate the user challenges posed by AutoML and automated data science, discuss the current state of the art, and propose directions for new research. More specifically, this seminar: - articulates the state of the art in AutoML and automated data science for supporting the provenance of decision making, - describes the challenges that data scientists and domain experts face when interfacing with automated approaches to make sense of an automated decision, - examines the interface between data-centric, model-centric, and user-centric models of provenance and how they interact with automated techniques, and - encourages exploration of human-centered approaches; for example leveraging visualization.
Citation
Anamaria Crisan,
Lars Kotthoff,
Marc
Streit,
Kai Xu
Human-Centered Approaches for Provenance in Automated Data Science (Dagstuhl Seminar 23372)
Dagstuhl Reports,
13(9):
116-136, doi:10.4230/DagRep.13.9.116, 2024.
BibTeX
@article{2024_human_prov_automl, title = {Human-Centered Approaches for Provenance in Automated Data Science (Dagstuhl Seminar 23372)}, author = {Anamaria Crisan and Lars Kotthoff and Marc Streit and Kai Xu}, journal = {Dagstuhl Reports}, publisher = {Schloss Dagstuhl -- Leibniz-Zentrum für Informatik}, address = {Dagstuhl, Germany}, doi = {10.4230/DagRep.13.9.116}, url = {https://drops.dagstuhl.de/entities/document/10.4230/DagRep.13.9.116}, volume = {13}, number = {9}, pages = {116-136}, month = {3}, year = {2024} }