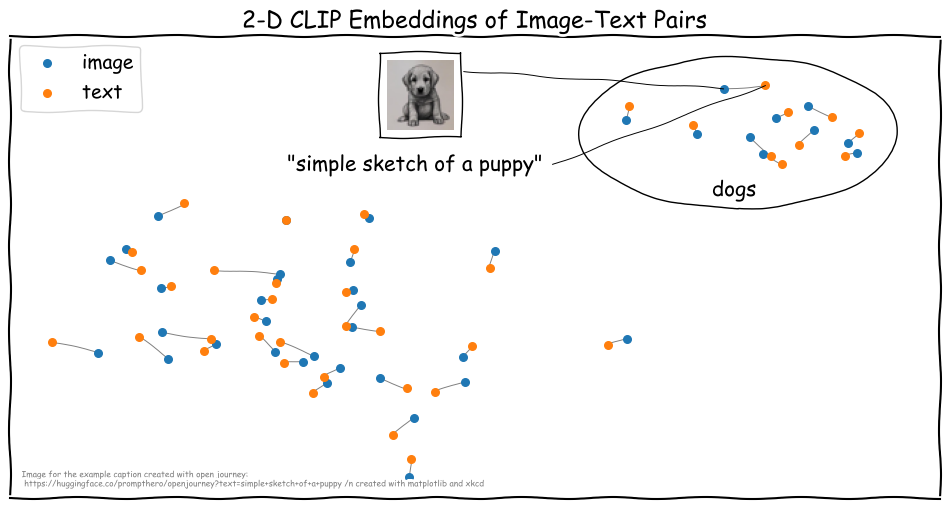
Abstract
Contrastive Language Image Pre-training (CLIP) and variations of this approach like CyCLIP, or CLOOB are trained on image-text pairs with a contrastive objective. The goal of contrastive loss objectives is to minimize latent-space distances of data points that have the same underlying meaning. We refer to the particular cases of contrastive learning that CLIP-like models perform as multi-modal contrastive learning because they use two (or more) modes of data (e.g., images and texts) where each mode uses their own encoder to generate a latent embedding space. More specifically, the objective that CLIP is optimized for minimizes the distances between image-text embeddings of pairs that have the same semantic meaning while maximizing the distances to all other combinations of text and image embeddings. We would expect that such a shared latent space places similar concepts of images and texts close to each other. However, the reality is a bit more complicated.
Citation
Christina
Humer,
Vidya Prasad,
Marc
Streit,
Hendrik Strobelt
Understanding and Comparing Multi-Modal Models: Exploring the Latent Space of CLIP-like Models (CLIP, CyCLIP, CLOOB) Using Inter-Modal Pairs
6th Workshop on Visualization for AI Explainability,
2023.
Best Submission Award at VISxAI 2023
BibTeX
@article{, title = {Understanding and Comparing Multi-Modal Models: Exploring the Latent Space of CLIP-like Models (CLIP, CyCLIP, CLOOB) Using Inter-Modal Pairs}, author = {Christina Humer and Vidya Prasad and Marc Streit and Hendrik Strobelt}, journal = {6th Workshop on Visualization for AI Explainability}, url = {https://jku-vds-lab.at/amumo}, month = {October}, year = {2023} }
Acknowledgements
This work was funded by the Austrian Marshall Plan Foundation under the Marshall Plan Scholarship, the Austrian Science Fund under grant number FWF DFH 23–N, and under the Human-Interpretable Machine Learning project (funded by the State of Upper Austria). The project was conducted during a research visit at the MIT-IBM Watson AI Lab in Cambridge, MA. We would like to thank Elisabeth Rumetshofer for her feedback on CLOOB and its analysis.