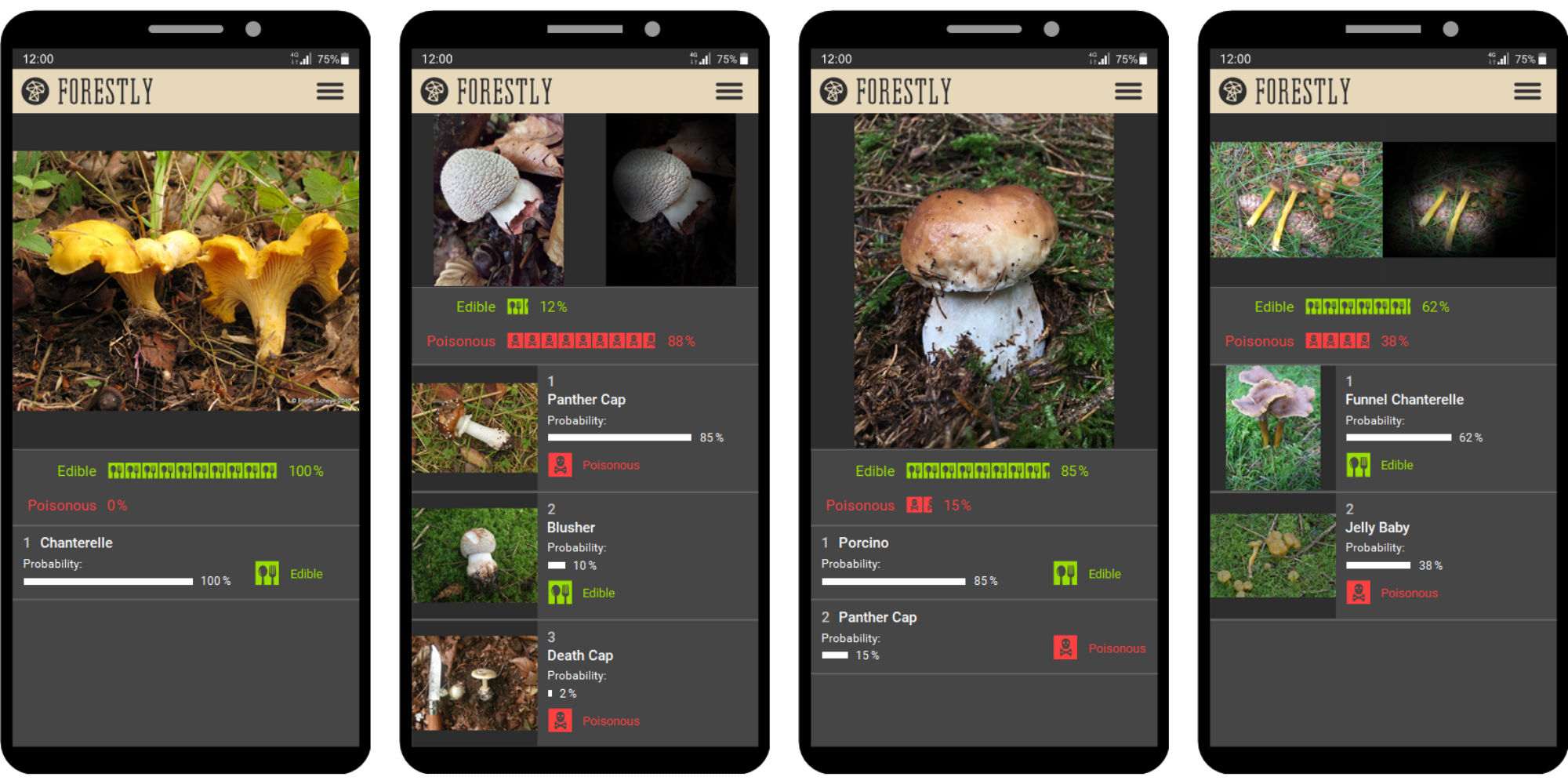
Abstract
Understanding the recommendation of an artificially intelligent (AI) assistant for decision-making is especially important in high-risk tasks, such as deciding whether a mushroom is edible or poisonous. To foster user understanding and appropriate trust in such systems, we tested the effects of explainable artificial intelligence (XAI) methods and an educational intervention on AI-assisted decision-making behavior in a 2x2 between subjects online experiment with N = 410 participants. We developed a novel use case in which users go on a virtual mushroom hunt and are tasked with picking only edible mushrooms but leaving poisonous ones. Additionally, users were provided with an AI-based app that shows classification results of mushroom images. For the manipulation of explainability, one subgroup additionally received attribution-based and example-based explanations of the AI's predictions, and for the educational intervention one subgroup received additional information on how the AI worked. We found that the group with explanations outperformed the group without explanations and showed more appropriate trust levels. Contrary to our expectation, we did not find effects for the educational intervention, domain-specific knowledge, or AI knowledge on performance. We discuss practical implications and introduce the mushroom-picking task as a promising use case for XAI research.
Citation
Benedikt Leichtmann,
Christina
Humer,
Andreas
Hinterreiter,
Marc
Streit,
Martina Mara
Effects of Explainable Artificial Intelligence on trust and human behavior in a high-risk decision task
Computers in Human Behavior,
139:
107539, doi:10.1016/j.chb.2022.107539, 2023.
BibTeX
@article{, title = {Effects of Explainable Artificial Intelligence on trust and human behavior in a high-risk decision task}, author = {Benedikt Leichtmann and Christina Humer and Andreas Hinterreiter and Marc Streit and Martina Mara}, journal = {Computers in Human Behavior}, publisher = {Elsevier}, doi = {10.1016/j.chb.2022.107539}, url = {https://www.sciencedirect.com/science/article/pii/S0747563222003594}, volume = {139}, pages = {107539}, month = {February}, year = {2023} }
Acknowledgements
This work was funded by Johannes Kepler University Linz, Linz Institute of Technology (LIT), the State of Upper Austria, and the Federal Ministry of Education, Science and Research under grant number LIT-2019-7-SEE-117, awarded to MM and MS, the Austrian Science Fund under grant number FWF DFH 23–N, and under the Human-Interpretable Machine Learning project (funded by the State of Upper Austria).