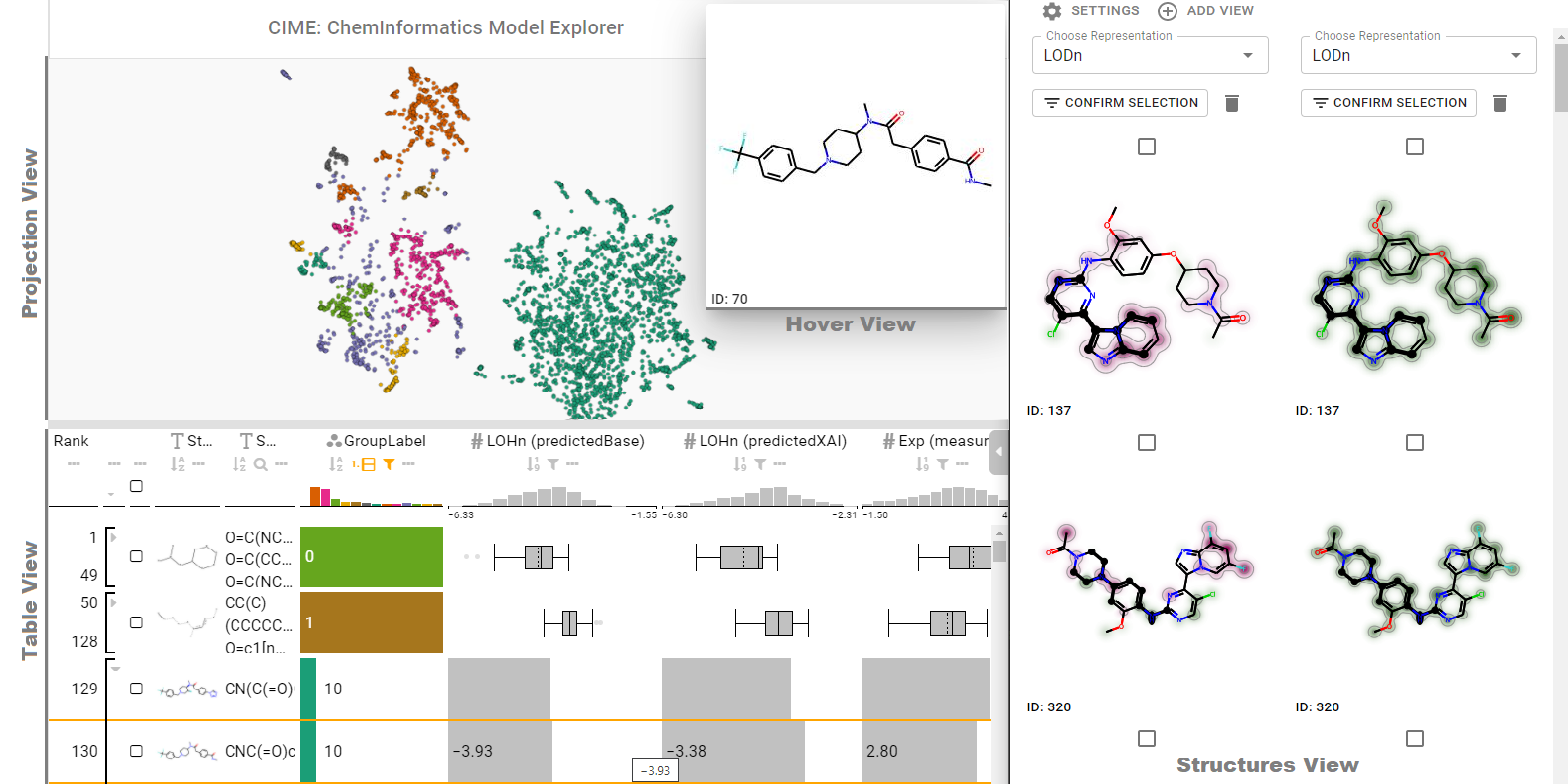
Abstract
The introduction of machine learning to small molecule research – an inherently multidisciplinary field in which chemists and data scientists combine their expertise and collaborate – has been vital to making screening processes more efficient. In recent years, numerous models that predict pharmacokinetic properties or bioactivity have been published, and these are used on a daily basis by chemists to make decisions and prioritize ideas. The emerging field of explainable artificial intelligence is opening up new possibilities for understanding the reasoning that underlies a model. In small molecule research, this means relating contributions of substructures of compounds to their predicted properties, which in turn also allows the areas of the compounds that have the greatest influence on the outcome to be identified. However, there is no interactive visualization tool that facilitates such interdisciplinary collaborations towards interpretability of machine learning models for small molecules. To fill this gap, we present CIME (ChemInformatics Model Explorer), an interactive web-based system that allows users to inspect chemical data sets, visualize model explanations, compare interpretability techniques, and explore subgroups of compounds. The tool is model-agnostic and can be run on a server or a workstation.
Citation
Christina
Humer,
Henry Heberle,
Floriane Montanari,
Thomas Wolf,
Florian Huber,
Ryan Henderson,
Julian Heinrich,
Marc
Streit
ChemInformatics Model Explorer (CIME): Exploratory Analysis of Chemical Model Explanations
Journal of Cheminformatics,
14(21):
doi:10.1186/s13321-022-00600-z, 2022.
BibTeX
@article{, title = {ChemInformatics Model Explorer (CIME): Exploratory Analysis of Chemical Model Explanations}, author = {Christina Humer and Henry Heberle and Floriane Montanari and Thomas Wolf and Florian Huber and Ryan Henderson and Julian Heinrich and Marc Streit}, journal = {Journal of Cheminformatics}, doi = {10.1186/s13321-022-00600-z}, url = {https://doi.org/10.1186/s13321-022-00600-z}, volume = {14}, number = {21}, year = {2022} }
Acknowledgements
This work was supported by the JKU Visual Data Science Lab and Bayer AG (HRB 48248). We thank Michael Koch for participating in the initiation of the project and for follow-up discussions; Michael Pühringer for reading the final version of the article and Moritz Heckmann for technical support.
Funding
This work was supported in part by Bayer AG, State of Upper Austria and the Austrian Federal Ministry of Education, Science and Research via the LIT - Linz Institute of Technology (LIT-2019-7-SEE-117), and the Austrian Science Fund (FWF DFH 23–N). TW and FH acknowledge funding from the Bayer AG Life Science Collaboration Project (“Machine Guided Compound Profiling”). HH, RH, FM and JH acknowledge funding from the Bayer AG Life Science Collaboration Project (“Explainable AI”).