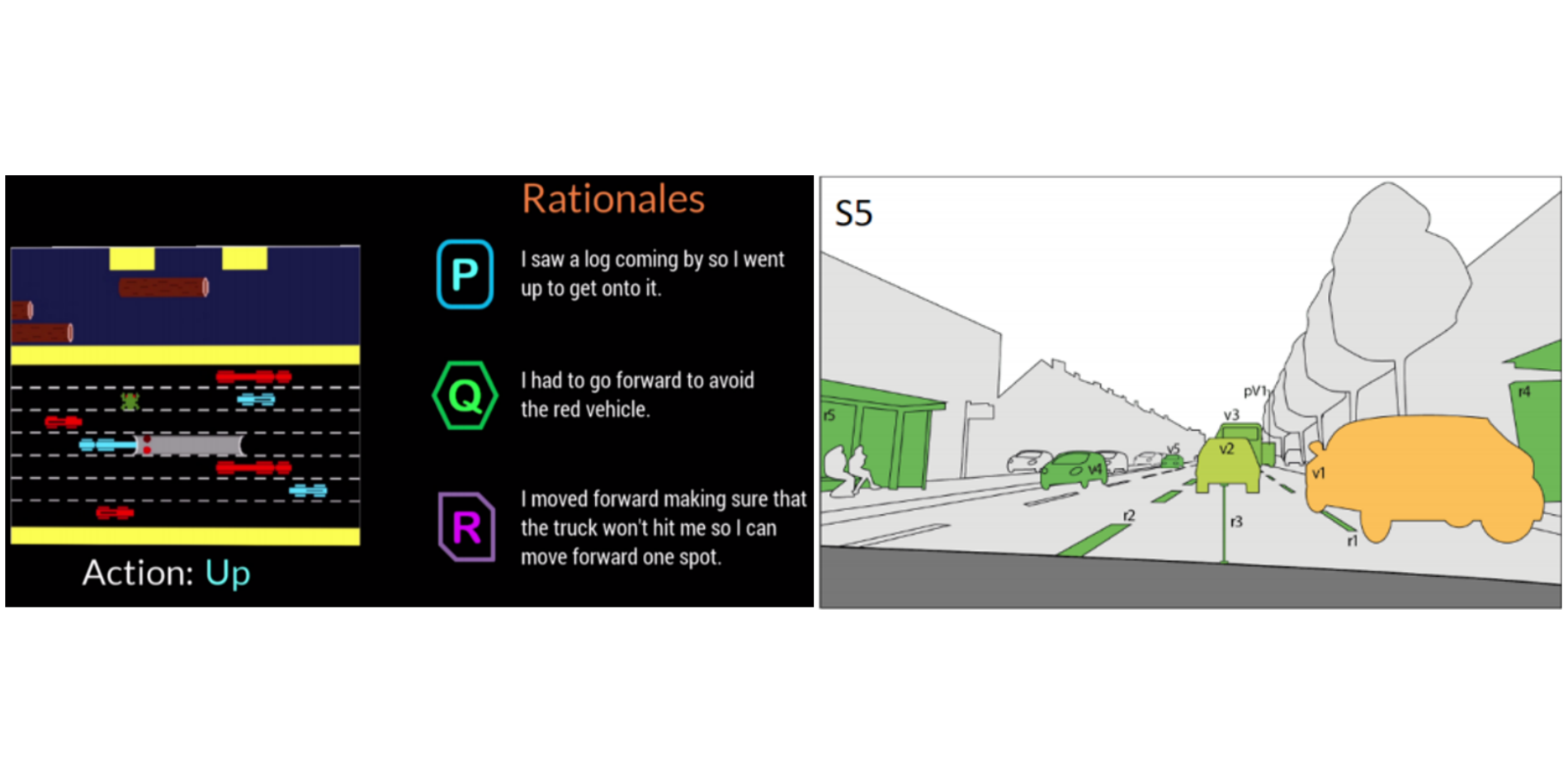
Abstract
The realm of Artificial Intelligence (AI)’s impact on our lives is far reaching – with AI systems proliferating high-stakes domains such as healthcare, finance, mobility, law, etc., these systems must be able to explain their decision to diverse end-users comprehensibly. Yet the discourse of Explainable AI (XAI) has been predominantly focused on algorithm-centered approaches, suffering from gaps in meeting user needs and exacerbating issues of algorithmic opacity. To address these issues, researchers have called for human-centered approaches to XAI. There is a need to chart the domain and shape the discourse of XAI with reflective discussions from diverse stakeholders. The goal of this workshop is to examine how human-centered perspectives in XAI can be operationalized at the conceptual, methodological, and technical levels. Encouraging holistic (historical, sociological, and technical) approaches, we put an emphasis on “operationalizing”, aiming to produce actionable frameworks, transferable evaluation methods, concrete design guidelines, and articulate a coordinated research agenda for XAI.
Citation
Uphol Ehsan,
Philipp Wintersberger,
Q. Vera Liao,
Martina Mara,
Marc
Streit,
Sandra Wachter,
Andreas Riener,
Mark O. Riedl
Operationalizing Human-Centered Perspectives in Explainable AI
Extended Abstracts of the 2021 CHI Conference on Human Factors in Computing Systems (CHI EA '21), (94)
1--6, doi:10.1145/3411763.3441342, 2021.
BibTeX
@inproceedings{, title = {Operationalizing Human-Centered Perspectives in Explainable AI}, author = {Uphol Ehsan and Philipp Wintersberger and Q. Vera Liao and Martina Mara and Marc Streit and Sandra Wachter and Andreas Riener and Mark O. Riedl}, booktitle = {Extended Abstracts of the 2021 CHI Conference on Human Factors in Computing Systems (CHI EA '21)}, publisher = {Association for Computing Machinery, New York, NY, United States}, editor = {Yoshifumi Kitamura, Aaron Quigley, Katherine Isbister, Takeo Igarashi}, doi = {10.1145/3411763.3441342}, url = {https://doi.org/10.1145/3411763.3441342}, number = {94}, pages = {1--6}, month = {May}, year = {2021} }
Acknowledgements
This work is supported under the FH-Impuls program of the German Federal Ministry of Education and Research (BMBF) under Grant Number 13FH7I01IA (SAFIR). We are grateful to members of the Human-centered AI lab at Georgia Tech for their input during brainstorming of these ideas.