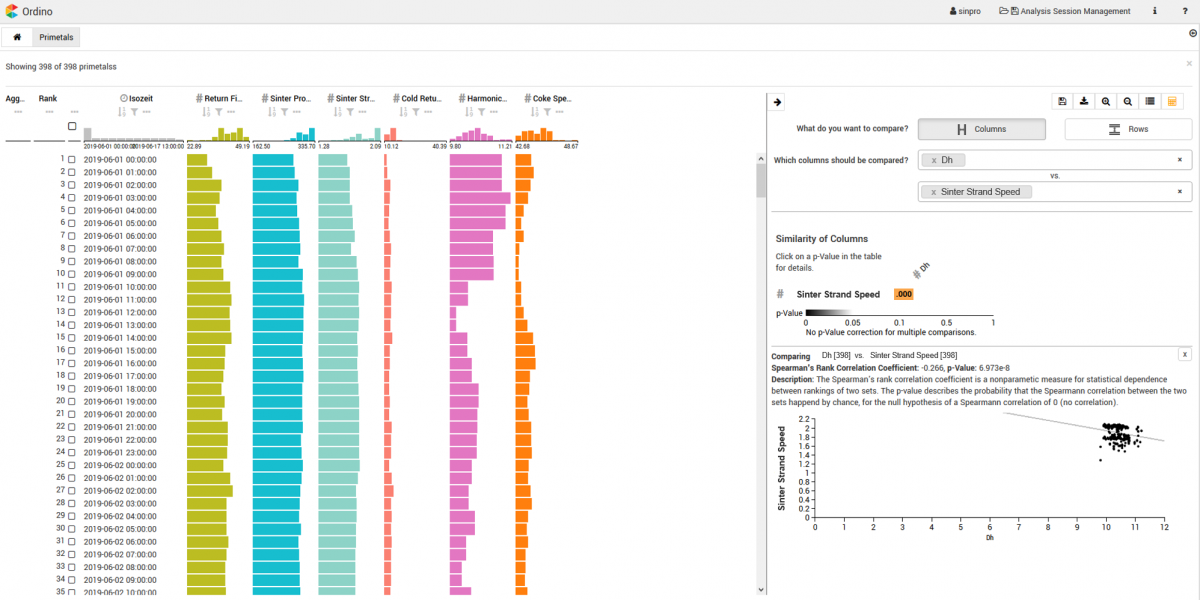
Abstract
Sintering is a complex production process where the process stability and product quality depend on various parameters. Building a forecasting model improves this process. Artificial intelligence (AI) approaches show promising results in comparison to current physical models. They are mostly considered black box models because of their hidden layers. Due to their complexity and limited traceability, it is difficult to draw conclusions for real sinter processes and improving the physical models in a running plant. This challenge is addressed by focusing on detecting causal links from AI-based forecasting models in order to improve the understanding of sintering and optimizing existing physical models.
Citation
Matej Vukovic,
Vaishali
Dhanoa,
Markus Jäger,
Conny
Walchshofer,
Josef Küng,
Petra Krahwinkler,
Belgin Mutlu,
Stefan Thalmann
A Forecasting Model-Based Discovery of Causal Links of Key Influencing Performance Quality Indicators for Sinter Production Improvement
Iron & Steel Technology,
doi:10.33313/380/218, 2021.
BibTeX
@article{, title = {A Forecasting Model-Based Discovery of Causal Links of Key Influencing Performance Quality Indicators for Sinter Production Improvement}, author = {Matej Vukovic and Vaishali Dhanoa and Markus Jäger and Conny Walchshofer and Josef Küng and Petra Krahwinkler and Belgin Mutlu and Stefan Thalmann}, journal = {Iron & Steel Technology}, doi = {10.33313/380/218}, year = {2021} }
Acknowledgements
This work has partially been supported by the FFG, Contract No. 854184: “Pro2Future is funded within the Austrian COMET Program Competence Centers for Excellent Technologies under the auspices of the Austrian Federal Ministry of Transport, Innovation and Technology, the Austrian Federal Ministry for Digital and Economic Affairs and of the Provinces of Upper Austria and Styria. COMET is managed by the Austrian Research Promotion Agency FFG.”