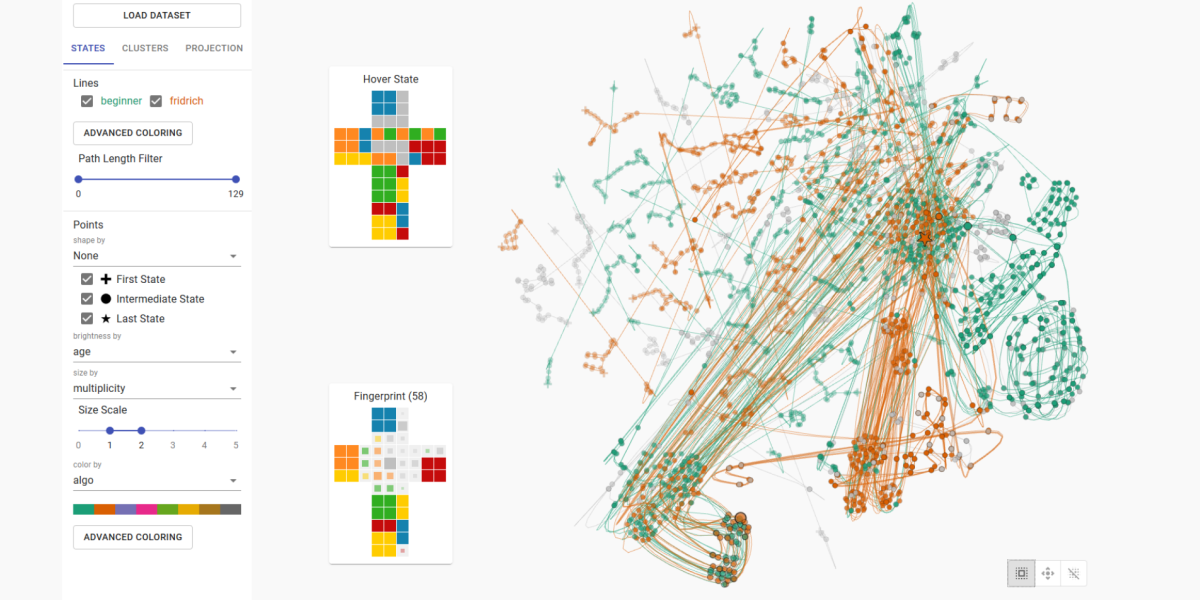
Abstract
In problem-solving, a path towards solutions can be viewed as a sequence of decisions. The decisions, made by humans or computers, describe a trajectory through a high-dimensional representation space of the problem. By means of dimensionality reduction, these trajectories can be visualized in lower-dimensional space. Such embedded trajectories have previously been applied to a wide variety of data, but analysis has focused almost exclusively on the self-similarity of single trajectories. In contrast, we describe patterns emerging from drawing many trajectories—for different initial conditions, end states, and solution strategies—in the same embedding space. We argue that general statements about the problem-solving tasks and solving strategies can be made by interpreting these patterns. We explore and characterize such patterns in trajectories resulting from human and machine-made decisions in a variety of application domains: logic puzzles (Rubik's cube), strategy games (chess), and optimization problems (neural network training). We also discuss the importance of suitably chosen representation spaces and similarity metrics for the embedding.
Citation
Andreas
Hinterreiter,
Christian A.
Steinparz,
Moritz Heckmann,
Holger
Stitz,
Marc
Streit
Projection Path Explorer: Exploring Visual Patterns in Projected Decision-Making Paths
ACM Transactions on Interactive Intelligent Systems,
11(3–4):
Article 22, doi:10.1145/3387165, 2021.
BibTeX
@article{2020_tiis_pathexplorer, title = {Projection Path Explorer: Exploring Visual Patterns in Projected Decision-Making Paths}, author = {Andreas Hinterreiter and Christian A. Steinparz and Moritz Heckmann and Holger Stitz and Marc Streit}, journal = {ACM Transactions on Interactive Intelligent Systems}, doi = {10.1145/3387165}, url = {https://dl.acm.org/doi/10.1145/3387165}, volume = {11}, number = {3–4}, pages = {Article 22}, year = {2021} }
Acknowledgements
This work was supported in part by the State of Upper Austria and the Austrian Federal Ministry of Education, Science and Research via the LIT – Linz Institute of Technology (LIT-2019-7-SEE-117), by the State of Upper Austria (Human-Interpretable Machine Learning), by the Austrian Research Promotion Agency (FFG851460), and by the Austrian Science Fund (FWF P27975-NBL).