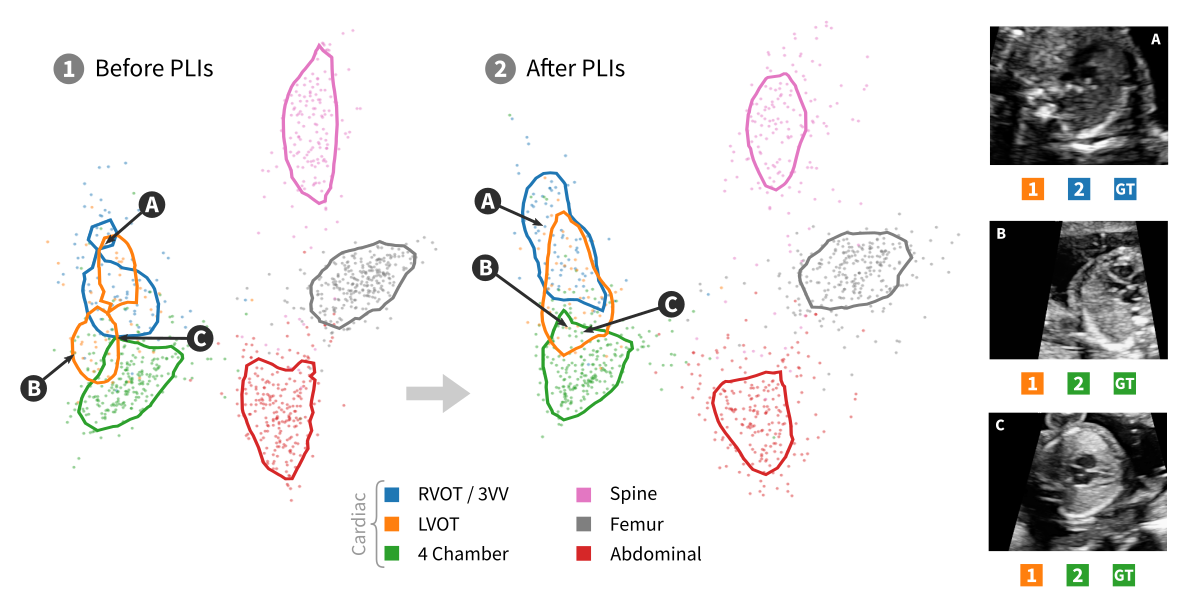
Abstract
High-dimensional latent representations learned by neural network classifiers are notoriously hard to interpret. Especially in medical applications, model developers and domain experts desire a better understanding of how these latent representations relate to the resulting classification performance. We present a framework for retraining classifiers by backpropagating manual changes made to low-dimensional embeddings of the latent space. This means that our technique allows the practitioner to control the latent decision space in an intuitive way. Our approach is based on parametric approximations of non-linear embedding techniques such as t-distributed stochastic neighbourhood embedding. Using this approach, it is possible to manually shape and declutter the latent space of image classifiers in order to better match the expectations of domain experts or to fulfil specific requirements of classification tasks. For instance, the performance for specific class pairs can be enhanced by manually separating the class clusters in the embedding, without significantly affecting the overall performance of the other classes. We evaluate our technique on a real-world scenario in fetal ultrasound imaging.
Citation
Andreas
Hinterreiter,
Marc
Streit,
Bernhard Kainz
Projective Latent Interventions for Understanding and Fine-tuning Classifiers
Interpretable and Annotation-Efficient Learning for Medical Image Computing. Proceedings of the 3rd Workshop on Interpretability of Machine Intelligence in Medical Image Computing (iMIMIC 2020),
12446:
13--22, doi:10.1007/978-3-030-61166-8_2, 2020.
Best Paper Award at iMIMIC 2020
BibTeX
@inproceedings{2020_imimic_projective-latent-interventions, title = {Projective Latent Interventions for Understanding and Fine-tuning Classifiers}, author = {Andreas Hinterreiter and Marc Streit and Bernhard Kainz}, booktitle = {Interpretable and Annotation-Efficient Learning for Medical Image Computing. Proceedings of the 3rd Workshop on Interpretability of Machine Intelligence in Medical Image Computing (iMIMIC 2020)}, publisher = {Springer}, url = {https://link.springer.com/chapter/10.1007%2F978-3-030-61166-8_2}, editor = {Cardoso, Jaime and others}, series = {Lecture Notes in Computer Science}, doi = {10.1007/978-3-030-61166-8_2}, volume = {12446}, pages = {13--22}, year = {2020} }
Acknowledgements
This work was supported by the State of Upper Austria (Human-Interpretable Machine Learning) and the Austrian Federal Ministry of Education,Science and Research via the Linz Institute of Technology (LIT-2019-7-SEE-117), andby the Wellcome Trust (IEH 102431 and EPSRC EP/S013687/1.)